Anyone who was around for the early days of the World Wide Web, before the Netscape IPO and the dotcom boom, knows that there was a strange quality to the medium back then – in many ways the exact opposite of the way the Web works today. It was oddly devoid of people. Tim Berners-Lee had conjured up a radically new way of organizing information through the core innovations of hypertext and URLs, which created a standardized way of pointing to the location of documents. But almost every Web page you found yourself on back in those frontier days was frozen in the form that its author had originally intended. The words on the screen couldn’t adapt to your presence and your interests as you browsed. Interacting with other humans and having conversations – all that was still what you did with email or usenet or dial-up bulletin boards like The Well. The original Web was more like a magic library, filled with pages that could connect to other pages through miraculous wormholes of links. But the pages themselves were fixed, and everyone browsed alone.
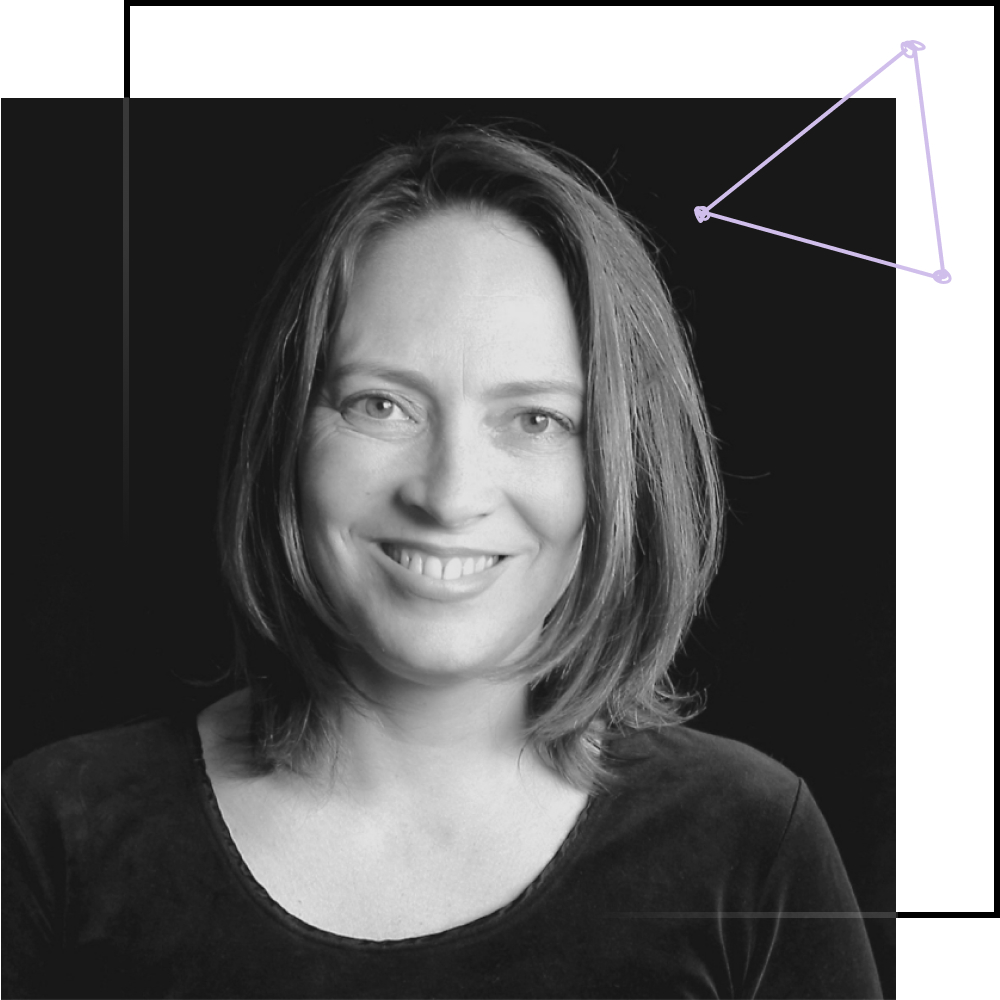
Developed in the late-70s, USENET was the more sociable sibling of email protocols that had emerged a few years earlier. USENET served for many years as the primary venue for distributed public dialogue, places where strangers could talk to one another in thematically-organized “newsgroups” like sci.physics and alt.politics. If you wanted to strike up a digital conversation with a specific friend or group of colleagues, email was your platform. But if you wanted to get up on a soapbox or find new friends who might share your interests, USENET was the way to go. It was also, it should be noted, a complete cesspit of porn and hate speech as well. The phrase “flame wars” had to be invented to describe the default tone of some newsgroups, and the first spam message in history appeared there. Though most online conversation ultimately shifted to the web — and then to social media — USENET newsgroups continue to be active to this day.
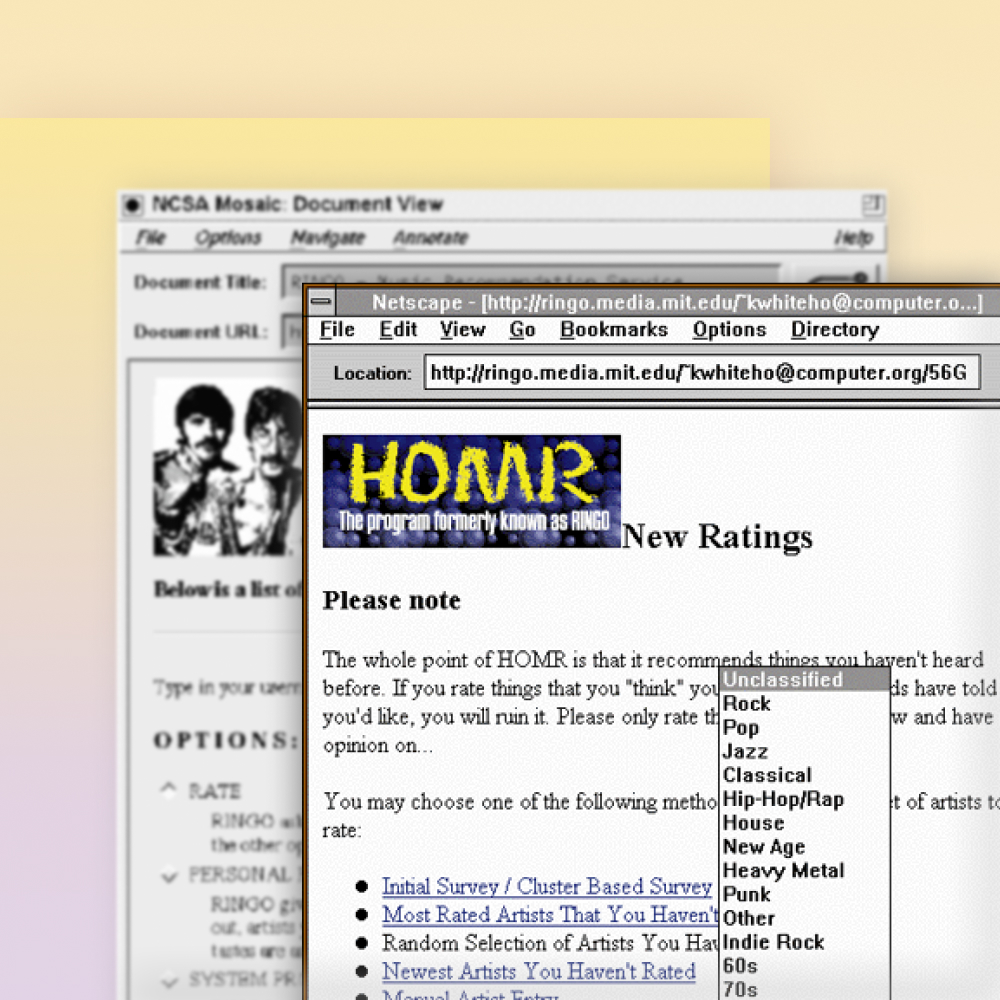
One of the first signs that the Web might eventually escape those confines arrived in the last months of 1994, with the release of an intriguing (albeit bare-bones) prototype called HOMR, short for the Helpful Online Music Recommendation service.
HOMR was one of a number of related projects that emerged in the early-to-mid-90s out of the MIT lab of the Belgian-born computer scientist Pattie Maes, projects that eventually culminated in a company that Maes co-founded, called Firefly. HOMR pulled off a trick that was genuinely unprecedented at the time: it could make surprisingly sophisticated recommendations of music that you might like. It seemed to be capable of learning something about you as an individual. Unlike just about everything else on the Web back then, HOMR’s pages were not one-size-fits all. They suggested, perhaps for the first time, that this medium was capable of conveying personalized information. Firefly would then take that advance to the next level: not just recommending music, but actually connecting you to other people who shared your tastes.
Maes called the underlying approach “collaborative filtering”, but looking back on it with more than two decades’ worth of hindsight, it’s clear that what we were experiencing with HOMR and Firefly was the very beginnings of a new kind of software platform that would change the world in the coming decades, for better and for worse: social networks.
Rise of the Software Agent
Maes was born in the early 1960s in Brussels, the child of a doctor and a dentist. “I always tell people, I was not the type of kid who took apart radios and built robots,” she says now, looking back on those early years. “I emphasize that because when I was growing up whenever I read an article a computer scientist—that's what they would say. But that wasn’t me. I was playing with Barbies—and Legos.”
Arriving as an undergrad at the Free University of Brussels during the late-1970s oil crisis, Maes initially gravitated towards a computer science major for entirely practical reasons. “There were no jobs for kids leaving college,” she says, “and though I wanted to either study architecture or biology, I eventually ended up choosing computer science, really for two reasons. I did realize that computers were going to be important in any domain, so I could still do biology or architecture in the future. But the other reason was purely practical: I’d definitely have a job when I graduated.” It wasn’t until she enrolled in a class on artificial intelligence that Maes found herself intellectually engaged with the material.
“AI was all about modeling human intelligence back then,” she recalls. “I thought: wow, this relates to people.” Within a few years she earned a PhD in Artificial Intelligence, and moved to the US to do post-graduate work at MIT, studying with AI pioneers like Rodney Brooks and Marvin Minsky. “I came to visit first for like two months and then for a year, and then the year became two years,” she says. The move was a bold one for more than just geographic reasons. In the late eighties, the extended AI Lab at MIT consisted of around forty scholars. Maes was the only woman in the entire group.
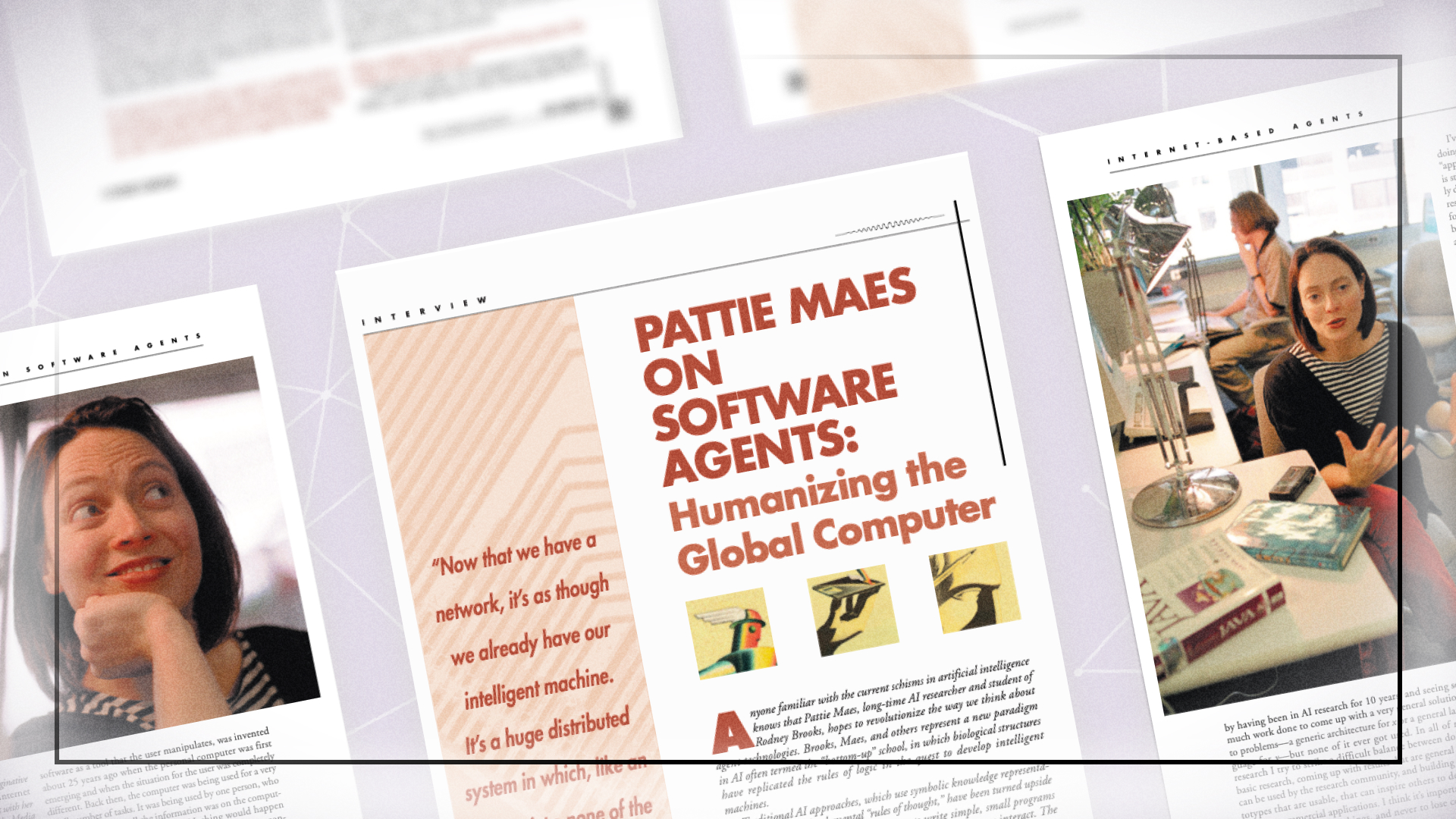
The late 80s and early 90s belonged to a longer period in AI research often referred to as the “AI Winter”—a frustrating stretch of time where the field appeared to make little progress, after an early wave of hype in the 60s and 70s. Ultimately, Maes came to believe that AI back then was “just creating intelligent systems for the sake of making more intelligent systems,” she says now. “I was always much more interested in helping people—thinking about how technology could help us with decision-making and communication and finding other people that we might want to talk to. Or how it could augment our memories.”
Artificial intelligence first emerged as a field through a flurry of thrilling developments in the 1950s. Stanford’s John McCarthy first coined the phrase in the mid-50s, and by the end of the decade, computers were learning how to play elemental games like checkers. The Perceptron, the first “neural net” modeled on the architecture of the human brain — the ancestor of modern AI superstars like GPT-3 — was developed in 1958. At the time, the rapid early progress in the field suggested that simulating open-ended intelligence and problem solving was within reach. Reporting on the launch of the Perceptron, The New Yorker claimed that the machine “was capable of original thought. Indeed, it strikes us as the first serious rival to the human brain ever devised.” But genuine “rivals” to the human brain ended up requiring far more computational power than the technology world possessed in the ensuing decades. The field went through a decades-long stretch without making serious progress—now known as the “AI winter”—until the 2010s, when the emergence of organizations like DeepMind and OpenAI finally began to deliver on the original vision.
Working with a handful of grad students in a lab she called the “Software Agents” group, Maes began exploring the ways that shared social information could generate helpful recommendations. “We started this work actually before browsers existed,” Maes says now, with a chuckle. The first iteration revolved around science fiction novels, and was entirely e-mail based. You sent off an email with the names of sci-fi books you liked, and the software emailed back some suggestions for further reading, based on your tastes. A student of Maes’ named Karl Feynman—son of legendary physicist Richard Feynman—created an e-mail recommendation system for music, called RINGO. When Feynman left MIT, another grad student, Upendra Shardanand, began working on the browser-based version, HOMR, under Maes’ supervision. “The whole idea was really to kind of simulate the joy of going to a record store and browsing,” he says now, looking back on that original project. “There was something brain-tickling about the whole thing. It was all about the joy of discovery and exploration.”
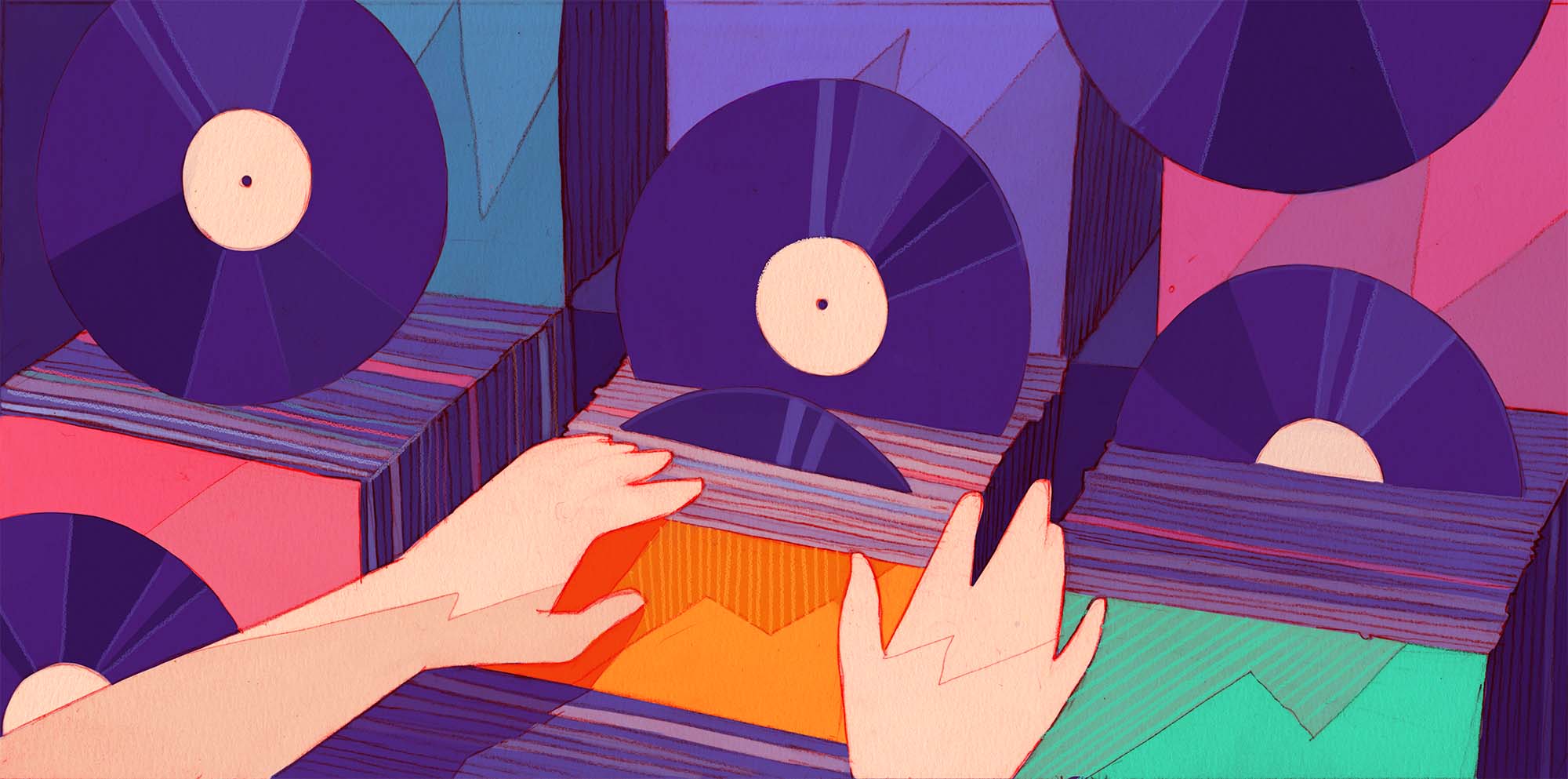
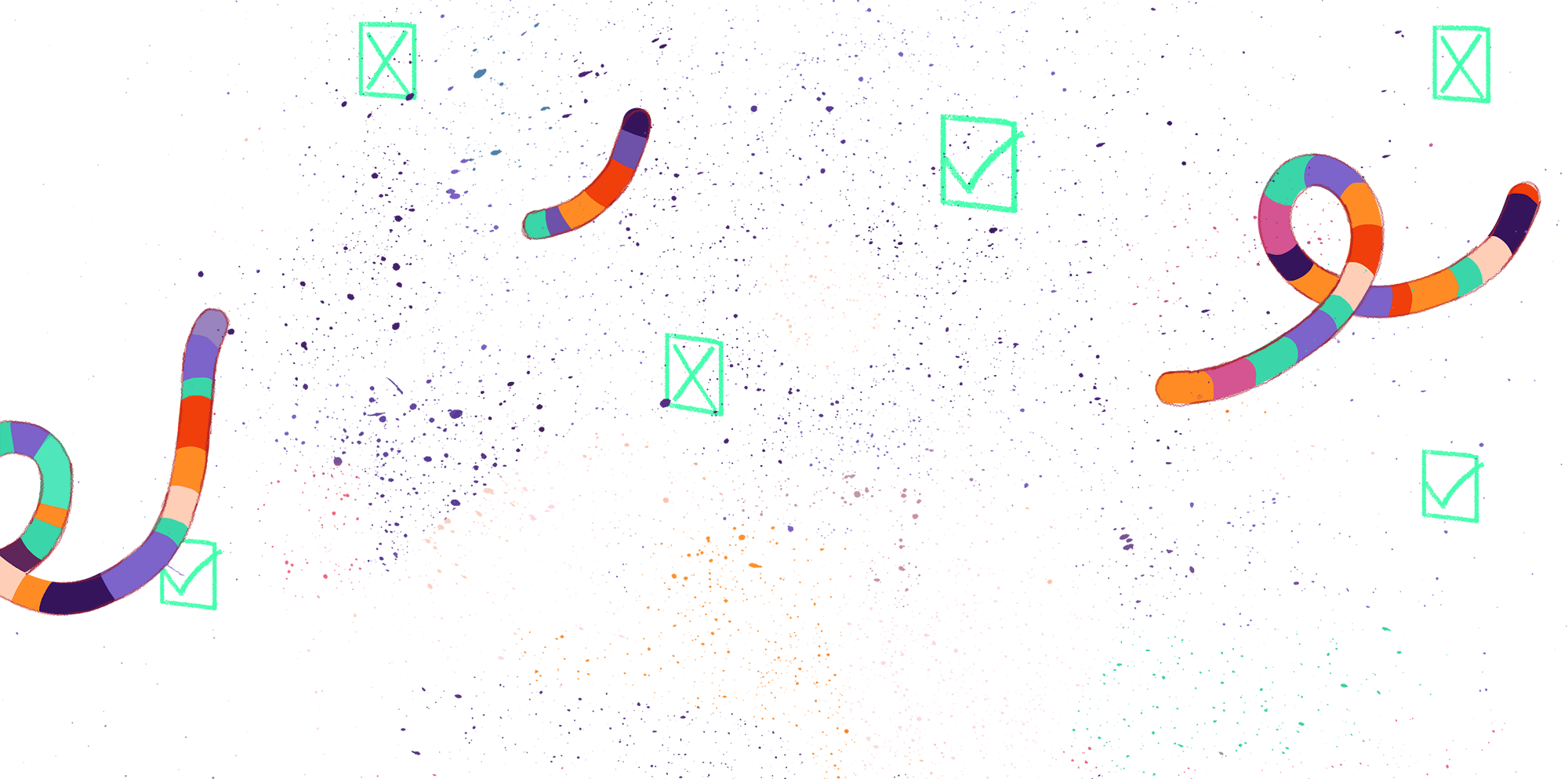
The interaction was simple: the software offered you a random sampling of artists to rate on a scale of 1-7: Arrested Development, Nirvana, Van Morrison, The Sex Pistols. Once you submitted the ratings, the software would recommend a list of albums that you might like, given your tastes. In a medium defined by static information, HOMR offered something different: it seemed, in a slightly uncanny way, to know a little bit about you, to have a feel for something as inchoate as musical taste. The page it served up with those music recommendations was composed on the fly – you weren’t just reading through the same archived page that a thousand other people had read. Some of the artists it recommended were invariably ones you already knew, and that was impressive enough given that you were getting these recommendations from an algorithm. But the real trick was getting a recommendation that you hadn’t come across before, a musician who did turn out to be in your wheelhouse once you tracked down one of their albums. HOMR wasn’t just a digital magic trick – it was surprisingly useful.

Part of the magic lay in the fact that HOMR’s aesthetic sensibility was not hard-coded in advance. A programmer somewhere didn’t just create a pre-existing database of artists, organized by explicitly defined sub-genres. Instead, the association between different artists—Pearl Jam with Soundgarden, Joni Mitchell with Neil Young—was emerging from the bottom-up out of thousands of ratings sets that had been submitted by early users. Over time, the software learned to detect clusters of musical taste in all that data, a kind of transitivity principle of taste. If you liked the Pet Shop Boys, and someone else liked the Pet Shop Boys and Simple Minds, there was a higher probability that you might like Simple Minds as well.
It wasn’t explicit in the software yet, but there was another latent implication that HOMR was predicated on—if you shared some overlapping set of cultural tastes or references with someone, then perhaps you might want to form a deeper connection with that person, that relationships between individuals could be organized and mapped statistically, using databases and computer algorithms.
Social Media origins
The conventional history dates the origins of social networking back to the late 1990s and early aughts, marked by the launch of services like SixDegrees.com, Friendster, and the photo-sharing site Flickr. But many of the core ideas that would shape the social media revolution—minus the advertising model that would ultimately cause so much trouble—originated with Maes’ research at the Media Lab well before then.
“A lot of what we did was model people and collect data,” Maes says now with a wry smile. “It sounds terrible now but we thought of this as a positive thing. We were a little bit naive I guess back then about how this would all be used in the long run. But we thought: well, if we know a little bit more about people and their interests, then we can help them.”
Originally, Maes called the technique social filtering. “But then somebody said ‘social filtering?—that sounds like Nazis.’” For a while, they tried adding the word “information” to the phrase to make it more palatable: “social information filtering.” But eventually Maes settled on a new name, one that briefly became a catchphrase of mid-90s Internet culture: collaborative filtering.

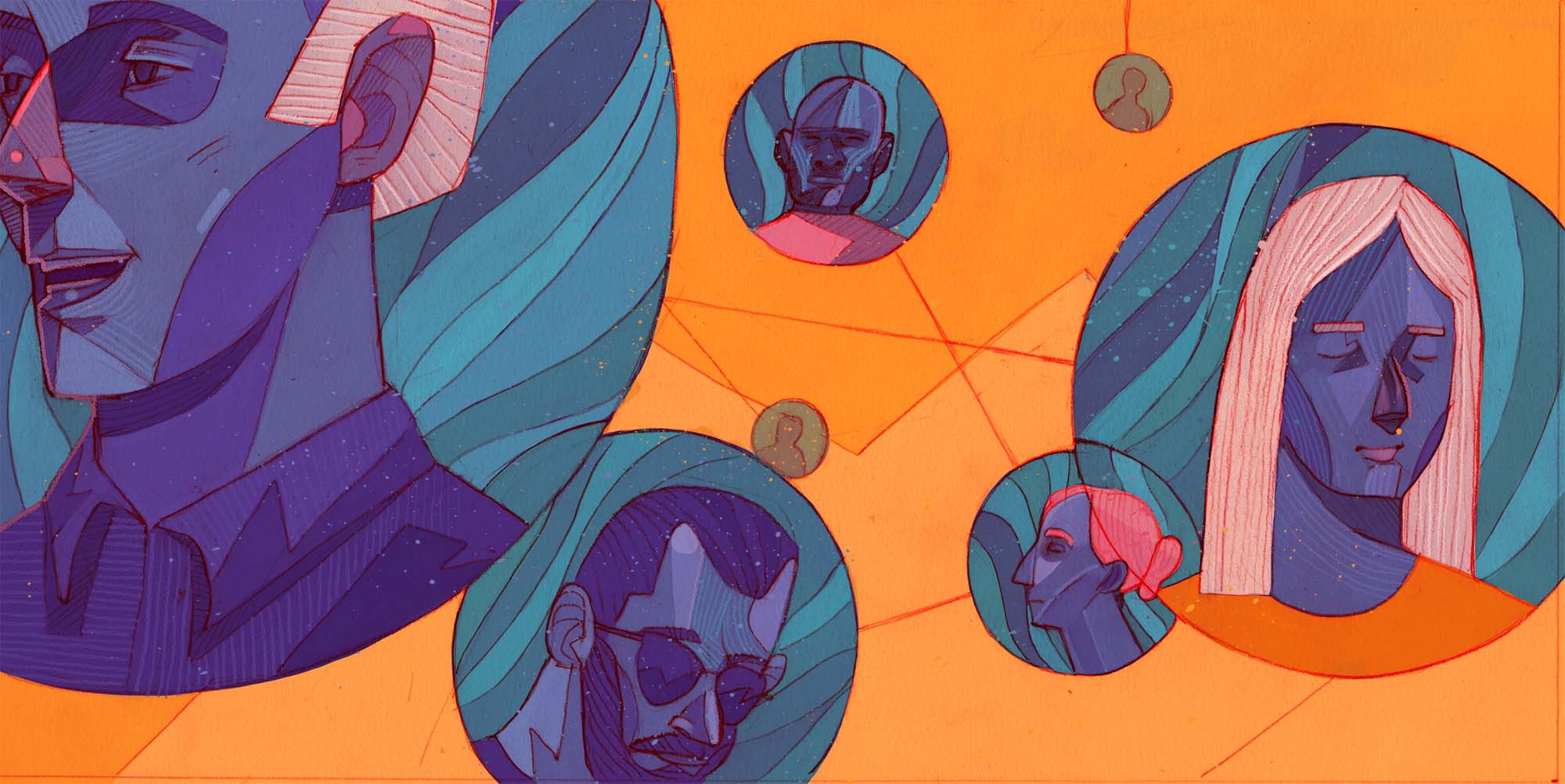
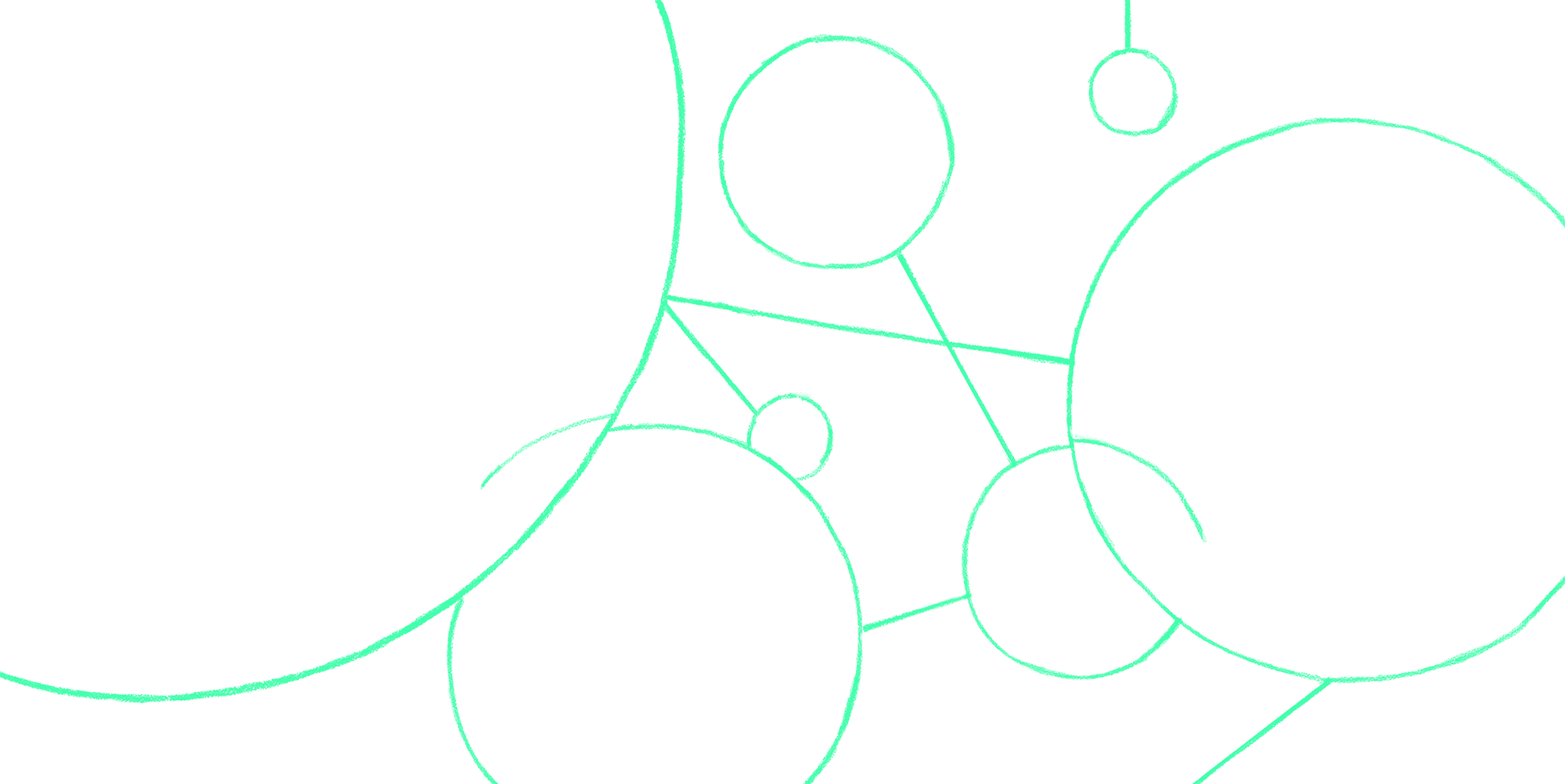
A paper Maes published in 1995, co-authored with Shardanand, laid out the approach in a clear language, free of the usual jargon of academic prose. “We need technology to help us wade through all the information to find the items we really want and need, and to rid us of the things we do not want to be bothered with.” You could sift through that information through traditional approaches like keyword filtering, but keywords were useless when trying to make more subtle assessments, like the ones at play when we like or dislike certain kinds of music. Other researchers were exploring automated ways of detecting meaning in text documents, using approaches like latent semantic indexing. But even if those techniques might be able to detect connections between articles online, they would be useless with other forms of media. Collaborative filtering took a different approach. As Maes and Shardanand wrote in the 1995 paper, the technique “essentially automates the process of ‘word-of-mouth’ recommendations: items are recommended to a user based upon values assigned by other people with similar taste. The system determines which users have similar taste via standard formulas for computing statistical correlations.” (The paper has now been cited almost five thousand times in other scholarly papers that followed in its wake.)
“Pattie back then—like Pattie now—as an academic advisor was really a zenmaster,” Shardanand says. “She was thoughtful, really listened to you, and had very insightful reactions.”
From Firefly to FaceMash
Before long, Maes and her students realized that collaborative filtering was useful for much more than simply recommending new artists or novels; once you’d given the computer a sense of your personal interests—and your connections to other people—all sorts of new possibilities emerged. “You can tell people how unique their interests are, like how rare are the books that they’re interested in,” Maes explains. “Or: who are the other people who like the same books or the same music that you like?”
What Maes’ research began to suggest was the possibility of organizing information around people: their likes and dislikes, their interests, their social circles. This seems obvious to us now, given that some of the most valuable companies in the world are predicated on this model, but in the mid-nineties Maes had a hard time convincing anyone that this could be a viable platform for a business.
Ultimately Maes and a team of students from MIT—including Sharpandra as CTO and a recent Harvard Business School grad named Nick Grouf as CEO—decided to take matters into their own hands and start a company themselves. “The whole idea of the Media Lab was always that we do the research and then these big companies take what we invent and commercialize it,” Maes says. “But [the big companies] weren’t doing that or they weren’t ready for it. So we started Firefly.”